The Journal of Clinical and Preventive Cardiology has moved to a new website. You are currently visiting the old
website of the journal. To access the latest content, please visit www.jcpconline.org.
Basic Research for Clinicians
2 x 2 Contingency Table: Fisher’s Exact Test
Volume 3, Apr 2014
Padam Singh, PhD, Gurgaon, India
J Clin Prev Caridiol. 2014;3(2):66-70
Background
In medical research, a new drug/ intervention is generally compared with standard treatment and outcomes assessed in terms of cured or not cured, and occurrence and non-occurrence of side effects etc. These variables are generally dichotomous i.e. Involves only two possible values. The data is generally displayed in a 2 x 2 contingency table that shows the frequencies of occurrence of all combinations of the levels of two dichotomous variables. In a sample of size n, a schematic form of such a table is given below
Table 1: A 2 × 2 contingency table.

Table 1: A 2 × 2 contingency table.

In the above contingency table it is customary to use rows for treatments and columns for outcomes. The margins in the contingency table are fixed, either by design or for the purposes of the analysis. For example, in a randomized trial in which the number of subjects to be randomized to each treatment group has been specified, the row margins would be fixed. On the other hand in a retrospective study, where one might sample cases
(diseased persons) and controls (non–diseased), and then record the presence or absence of some exposure, it would be the column margins that are fixed. However,
in a random sample, in which each subject sampled is cross–classified by two attributes none of the margins would be fixed.
A research question of interest is whether the attributes in the contingency table are associated or independent of each other. The null hypothesis would be that there is no association or there is no difference in proportions. Twomethods of analysis are available for this set-up.
i) Proportion tests based on Gaussian distribution
ii) Chi-square tests based on contingency table
For large samples both tests are equivalent and give identical results. However for small samples the analysis requires special treatment. This paper highlights the issues dealing with small numbers in 2 X 2 contingency table and suggests the appropriate procedure to be used with suitable illustrations.ii) Chi-square tests based on contingency table
Chi-square tests
Karl Pearson (1900) suggested use of test statistic as under:


For the example 1 considered above the Chi-square value with continuity correction works out as 2.25. The p value for this is 0.13361440. Contrary to the usual Chisquare test the results indicate no statistically significant difference. For example 2 the Chi-square value is 0.0173 and for this the p value works out as 0.0.8953. Thus the results after Yates correction for continuity are far different from the results of usual Chi-square tests.
It is important to mention that the Yates’ continuity correction is designed to make the approximation better. A comparison of binomial distribution (n =12 Π=0.5) with the approximating normal distribution is presented to illustrate the need for continuity correction for small ‘n’ When either nΠ or n-nΠ (Π being the binomial probability) are below 10, the accuracy of hypothesis test on the normal approximation can be improved by the introduction of a continuity correction. The continuity correction adjusts the numerator of the test statistic so that there is a closer fit. This is illustrated for observing 9 or more events out of 12 with probability, Π=0.5 in Table-2 below.

This test statistic under the null hypothesis follows a Chi-square (χ2) distribution with one degree of freedom.
Following graph presents a comparative picture of Chi square distribution with 1, 5 and 10 degrees of freedom.
Evidently the shape of Chi-square distribution with one degree of freedom is distinctly different with those of
higher degrees of freedom and hence demands special treatment in the statistical analysis.

Consider the following examples:
Example 1:


Consider the following examples:
Example 1:

Chi-Square = 4.000, DF = 1, P-Value = 0.046
The resulting p-value, 0.046, from the x2 test indicates that there is a statistically significant difference i.e. the
cure rate is higher for new drug as compared to the standard drug (at α = 0.05 level).
Example 2:


Chi-Square = 0.1931, DF = 1, P-Value = 0.6603
The resulting p-value 0.6603 indicates that the difference in the proportion of side effects in two drugs is not
statistically significant.
In the following sections, we shall examine whether the interpretation will undergo any change if other methods
are used for analysis.
Yates’s continuity correction
Yates (1934) argued that in case of small samples the χ2 distribution gives only approximate estimates of the
discrete probabilities associated with frequency data, and thus the p–values based on Pearson’s χ2 statistic
will generally underestimate the true p–values. In view
of this, Yates suggested that χ2 should be corrected for continuity and proposed the corrected test statistic as
under

For the example 1 considered above the Chi-square value with continuity correction works out as 2.25. The p value for this is 0.13361440. Contrary to the usual Chisquare test the results indicate no statistically significant difference. For example 2 the Chi-square value is 0.0173 and for this the p value works out as 0.0.8953. Thus the results after Yates correction for continuity are far different from the results of usual Chi-square tests.
It is important to mention that the Yates’ continuity correction is designed to make the approximation better. A comparison of binomial distribution (n =12 Π=0.5) with the approximating normal distribution is presented to illustrate the need for continuity correction for small ‘n’ When either nΠ or n-nΠ (Π being the binomial probability) are below 10, the accuracy of hypothesis test on the normal approximation can be improved by the introduction of a continuity correction. The continuity correction adjusts the numerator of the test statistic so that there is a closer fit. This is illustrated for observing 9 or more events out of 12 with probability, Π=0.5 in Table-2 below.
Table-2: Comparisons of the different methods of probability of observing 9 or more events, when n=12 and Π=0.5.

Source: Kirkwood BR, Sterne JAC. Medical Statistics, 2nd edition. Oxford: Blackwell Science Ltd; 2003, Page 146 The above table shows that incorporating a continuity correction and calculating the area under the normal

Source: Kirkwood BR, Sterne JAC. Medical Statistics, 2nd edition. Oxford: Blackwell Science Ltd; 2003, Page 146 The above table shows that incorporating a continuity correction and calculating the area under the normal
curve above 8.5 gives a close approximation to the exact binomial probability of observing 9 events or more. On
contrast the area of the normal curve above 9 is not a good approximation.
The same is presented graphically as under:

There are several issues related to Yates’s correction.


There are several issues related to Yates’s correction.
- The p–values obtained with the continuity correction are much less accurate when the binomial probabilityΠ is substantially away from 0.5.
- Yates’s correction is more appropriate only for one– sided tests, as it is based on a comparison betweenthe observed contingency and the next strongest contingency in the same direction. For two–sided tests, the statistic involves an over correction. Yates’s correction is systematically conservative when carrying out two–sided tests.
Fisher’s Exact Test
When the row and column margins in 2 X 2 contingency table are fixed, either by design or for the analysis, independence of attributes can be tested using Fisher’s exact test. This test is based on the hyper-geometric
distribution. The 2 X 2 contingency table can be presented as under:

The computation of probability for the contingency table with given cell frequencies using hyper-geometric distribution is as under
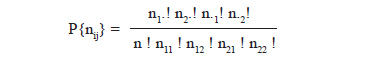
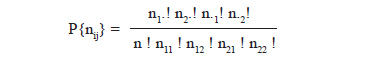
Computation of p values using Fisher’s Exact Test
To start with for given marginal totals all possible two way tables are generated which has desired marginal
totals. Thereafter, using the smallest marginal total the table for each ordered pair of integers with that sum is
created. The 2 X 2 contingency table for each of these ordered pairs are then completed. The probability for each contingency table is computed using hypergeometric distribution.
For a one tailed test n11 is compared with its expected value computed as
Corresponding row total X Corresponding column total
___________________________________________________
Grand Total
___________________________________________________
Grand Total
n11 exceeds its expected value then p value is the sum of the probabilities of all n11 more than equal to the expected value. Alternatively, if n11 is less than its expected value then p value is the sum of the probabilities of all n11 less than equal to the expected value.
The computation of p value for the two examples is explained as under:
Illustration 1: Following table presents the expected frequencies along with the observed frequencies for
example 1
Table 3: Comparison of observed and expectedfrequencies


It is observed that marginal totals are 9, 9 and 12, 6
Fisher’s exact test considers all possible cell combinations that would result in the marginal frequencies (namely 9, 9 and 12, 6).
Fisher’s exact test considers all possible cell combinations that would result in the marginal frequencies (namely 9, 9 and 12, 6).
The hyper-geometric probability distribution is used to compute the probability of the observed results. In
addition to the table of Example 1, other tables that will be consistent with the marginal frequencies of 9, 9
and 12, 6, along with their associated probabilities, are shown in Table 4.
Table 4: Computation of probability for different 2 X 2 tables corresponding to desired marginal totals.

Table 4: Computation of probability for different 2 X 2 tables corresponding to desired marginal totals.

Source: When To Use Fisher’s Exact Test by Keith M. Bower, M.S.
To compute Fisher’s exact test results, look at the tables with probabilities less than or equal to the probability of the observed results (0.061085972). They are highlighted with an *. Add these probabilities together, along with the probability of the observed results, to obtain the p-value for the test. This resulting p-value is 0.13122. Therefore, one would fail to reject the null hypothesis of independence at α = 0.05 level. This also contradicts
the results from the χ2 test and incidentally the p-value is close to that obtained using Yates correction.
Illustration 2: Following table presents the expected frequencies along with the observed frequencies for example 2
Table 5: Comparison of observed and expected frequencies
.jpg)
Illustration 2: Following table presents the expected frequencies along with the observed frequencies for example 2
Table 5: Comparison of observed and expected frequencies
.jpg)
Assuming that the marginal totals are fixed, all possible two way tables which have those marginal totals are worked out. Using the smallest marginal total and the tables for each ordered pair of integers with that sum are
created. Thereafter the 2x2 contingency tables for each of these ordered pairs are completed.
Since n2. = 4 is the smallest marginal total, we look for the following ordered pairs(n21, n22): (0, 4), (1, 3), (2, 2), (3, 1) and (4,0).
For each table the probability is computed as under:
Table 6: Computation of probability for different 2 X 2 tables of Example 2

Table 6: Computation of probability for different 2 X 2 tables of Example 2

Since the expected value is 7.6, which is less than the observed frequency 8 the P-value is .159197 + .409365 =.568562. Although the inference for Example 2 remains the same for test using χ2 test, Yates correction and Fisher’s Exact Test, the p-value is distinctly different.
Conclusion
The table below summarizes the comparison of p values for the two examples using different tests of significance

References

The highlights of conclusion are as under:
- χ2 test without Yates’s correction yields much lower p values.
- The p–values obtained using Yates’s correction tends to overestimate the true p–values.
- The Fisher’s test is exact because it uses the hypergeometric distribution.
- The results using Yates correction at times provide p-values close to the Fisher’s Exact test but it isnot the case always.
Importantly, the approximation using Pearson’s χ2 was necessary for the comparative simplicity of the calculations because the exact analysis of 2 X 2 contingency tables with the limited computing power available at the time was prohibitive in many cases. This is no longer the case. Yates’s correction is not necessary anymore since current software makes Fisher’s exact test computationally feasible. In view of this it is recommended to use Fisher’s Exact test for 2 X 2 contingency tables involving small numbers.
References
- Pearson, E.S. (1947) The choice of statistical tests illustrated on theinterpretation of data classed in a 2 × 2 table. Biometrika, 34, 139–167.
- Fisher, R.A. (1934) Statistical Methods for Research Workers. 5th Edition, Edinburgh: Oliver and Boyd.
- Yates, F. (1934) Contingency tables involving small numbers and the χ2 test. Journal of the Royal Statistical Society, Suppl.1, 217–235.
- Yates, F. (1984) Tests of significance for 2 × 2 contingency tables.Journal of the Royal Statistical Society Series A, 147, 426–463.
- Kirkwood BR, Sterne JAC. Medical Statistics, 2nd edition. Oxford:
Blackwell Science Ltd; 2003
- Why Publish with JCPC?
- Instructions to the Authors
- Submit Manuscript
- Advertise with Us
- Journal Scientific Committee
- Editorial Policy
Print: ISSN: 2250-3528
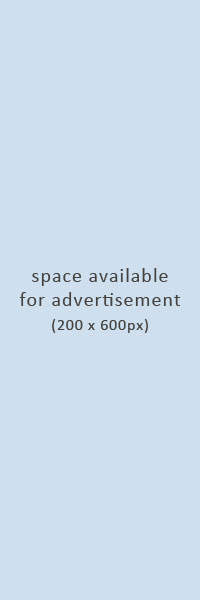